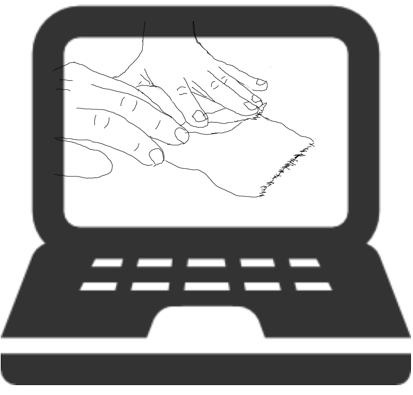
Designers may use ambiguous, contradictory, contextually driven, or even similar terms in describing physical material. Despite discipline-specific terms and taxonomies already defined, many of the terms are common to layman, everyday people.
If that is the case, crowdsourcing can be a promising way to source useful descriptions to both support material retail stores of all sizes and designers and hobbyists to be better informed on sought material.
What are the opportunities/challenges in crowdsourcing material description?
Role: Lead UX Researcher
Methods
Research Goal
How does material description differ between designers and everyday people?
How can crowdsourcing be used for more descriptions?
Research Problem
- How the following influence a participant’s understanding of materials remotely:
- Expertise
- Media representation of material (one image, multiple images, video, actual material)
Participants
20 who have 0+ experience, at least > 4 months experience, in building, making, and designing with fabric, from Virginia to various eastern metropolitan areas
20 who have 0 experience in building, making and designing with fabric
40 from the crowdsourcing community, who the state-of-the art posits, it’s the same as the 0 experience/everyday people
Research Design
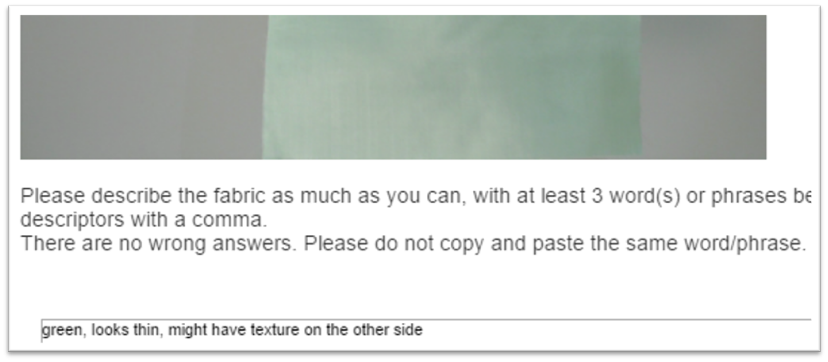
With the guidance of subject-matter experts, we designed images, multiple images, and videos that highlight the established properties of fabrics.
Since we had two main conditions (expertise and media), varying across 5 diverse
fabrics, we designed a within-subject study, within-subjects with respect to the fabrics and
the media conditions.
Task: Participants described digital versions, and actual fabric (for the in-person, non-crowdsourced participants) on 5 diverse fabrics. We also asked participants to reflect on similarities and differences.
Role
Lead UX researcher, designing and conducting all studies
Team: cross-disciplinary subject-matter experts
Duration
3 months
Tools
Qualtrics, Notepad, R, Amazon Turk, javascript
Skills
Survey design, Interviews, semantic analysis, crowdsourcing, statistical analysis
For in-person participants (0+ and 0 experienced participants
I used pre-questionnaires for prior experiences, surveys to input fabric descriptions, user preferences, and perceived comparisons, and interviews that asked them to reflect on perceived comparisons between the digital and actual fabric descriptions. I transcribed and conducted content analysis on the process and interview questions for higher-level insights.
For crowdsourced participants
In addition to the digital components of the in-person experiences, we made an initial qualifying question to assess the participants’ mastery of the English language, to maintain that all participants used the same language.
I transcribed all interviews data; using R, I converted all descriptions into its root words & analyzed the data by employing a non-parametric CHI^2 test to compare different audiences and representations between one another.
Scope/Limitations
Our in-person participants were located in Virginia, and to add more diversity in participants, I traveled to various northeastern metropolitan cities like New York, Boston and Philadelphia, recruiting in craft, fashion and designer venues.
Insights
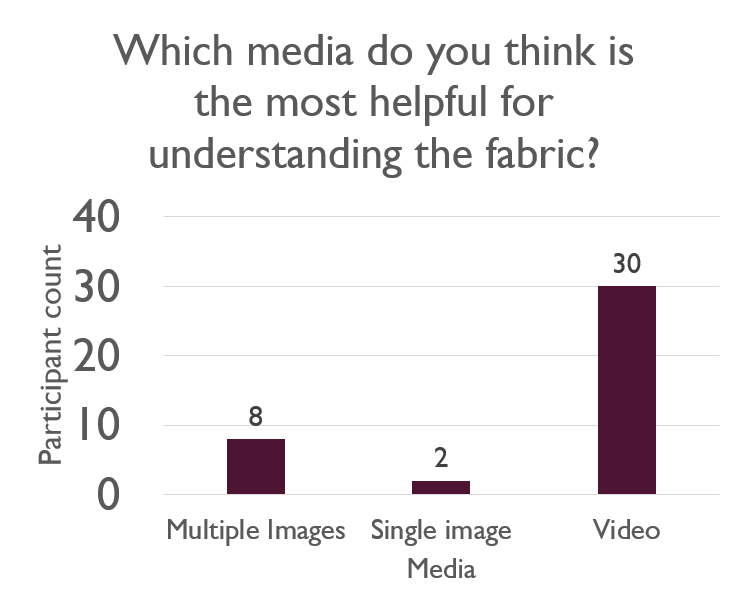
Video representation is the most informative way to show fabric remotely
Not only were videos the most preferred representation for the crowdsourcing community but when we found only one statistically significant different word(“synthetic” by video viewers) used between describing video and actual fabric, showing a large overlap in terminology.
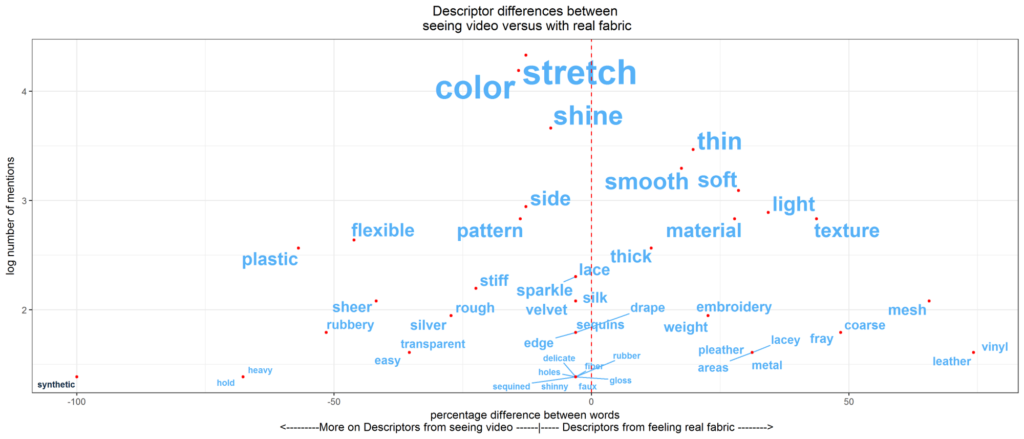
Few differences appeared between the crowdsourced and expert communities
In comparing expertise, crowdsourced community versus experienced community, we found very little statistical differences in the graph below. Experienced participants described with properties such as texture, drape, and stretch, but a myriad of other properties were used by both audiences.
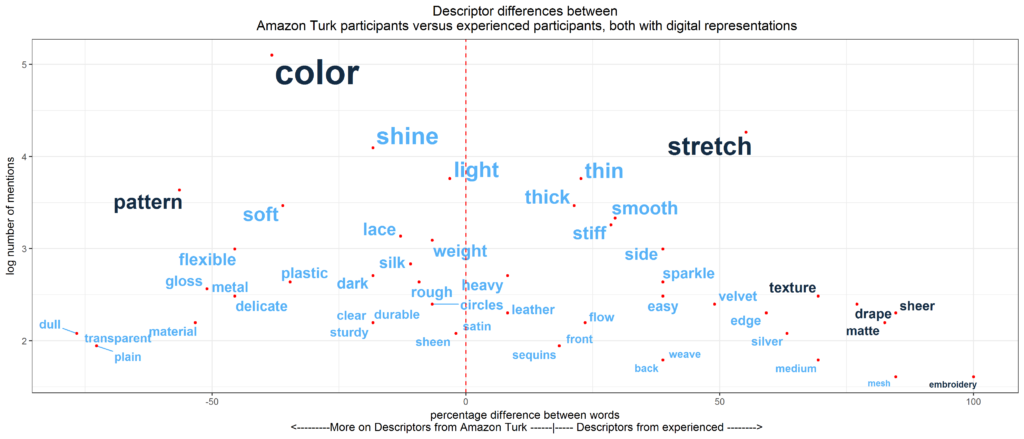
Implications for Design
Our results have direct applications to interface designers, marketplace sites, and especially businesses that cater to designers, hobbyists, and builders of craft.
Crowdsourcing is a promising venue to collect description
While we found a handful of expert-specific terms, the vast majority of descriptors used in this study were used by experts, non-experts and crowds alike. It shows crowdsourcing can obtain many descriptions with little time and resources.
Video is best for communicating materials remotely
Our preferences data and description data show video as the best substitute to getting a sense of material when unable to see it in person. By providing video, shoppers are best suited to be informed and order the best material with little surprises.
Best Practices: Use crowdsourcing with video for most descriptions, few experts with fabrics
To capture the best of all audiences, we suggest using crowdsourcing to get a healthy collection of descriptors on materials and rely on select experts to provide those few domain-specific terminologies.
Reflections
I was very surprised by how few experienced-specific terms we found; given that these groups may use the same words, (but potentially different interpretations), it amplified how important ambiguity can be in how viewers negotiate what the material would be like remotely, and made the final study of my thesis happen.
While I sought participants both in Virginia and in metropolitan areas like New York, Boston, and Philadelphia, I wish I could have gotten at least 20 more in-person participants for each category, so I can have the same number of participants in each group. While the current participant quantities helped us meet that goal, I am curious about what would happen if we doubled participants on all groups.